We’re Not in a Bubble—We’re at the Brink of Revolution
Every day I read another hot take claiming we’re in an AI bubble. These writers argue that Large Language Models cannot possibly lead to Artificial General Intelligence. And while they’ve got a point about the limitations of current systems, they’re catastrophically wrong about the bubble.
We’re not experiencing a bubble—we’re standing at the precipice of the most profound technological evolution in human history. The naysayers aren’t just mistaken; they’re blind to what’s unfolding right before our eyes. AI isn’t just getting started—it’s accelerating at a breathtaking pace that few truly comprehend. Research-backed projections support this view, suggesting we’re on a far steeper growth curve than most analysts realize.
I should clarify that I’m not an AI engineer or expert. I simply understand enough about how LLMs function to see what’s missing—the gap between our current technology and something genuinely revolutionary. And I’m going to show you how our current “lobotomized parrots” could transform into something far more profound.
How LLMs Actually Work
Before diving deeper, let’s review how today’s Large Language Models function. These systems are neural networks trained on vast amounts of text. During training, they adjust billions of “weights” (parameters) to minimize prediction errors, essentially learning to predict what words should follow other words.
The architecture behind modern LLMs is the transformer, which uses attention mechanisms to weigh the importance of different words for understanding context. The training process involves massive datasets and computing resources, with the model repeatedly adjusting its internal parameters to better predict language patterns.
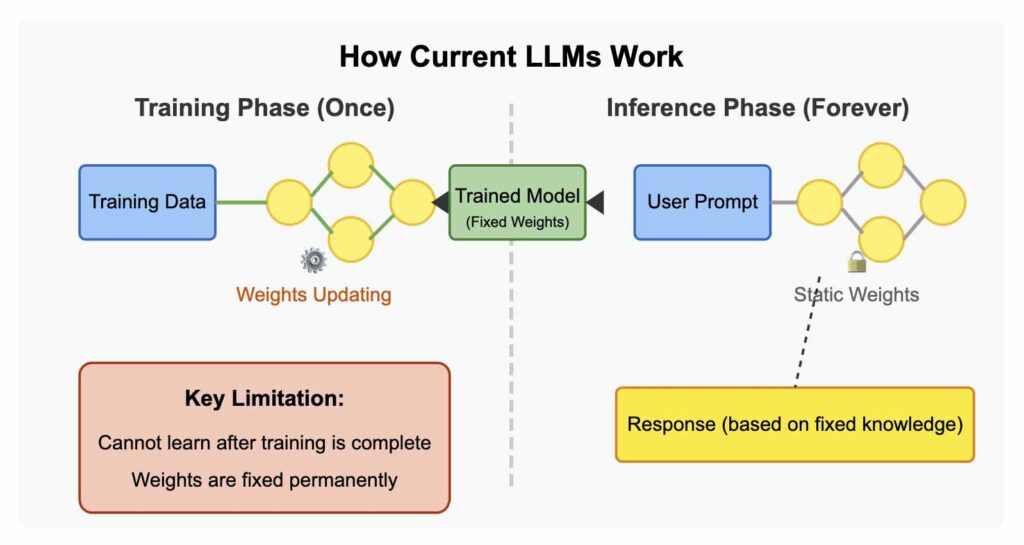
Why Current LLMs Are Limited—By Design
Despite their impressive capabilities, today’s LLMs are fundamentally limited—essentially functioning like sophisticated “lobotomized parrots.” They use neural networks that can only learn once, during their initial training phase, after which they can only infer based on that fixed knowledge base.
Once an LLM completes its training, it becomes incapable of learning anything truly new. The weights in its neural network become static. It transforms into an extraordinarily sophisticated word calculator—but still just a calculator, working with the fixed knowledge it was trained on.
The industry has developed clever workarounds to mitigate these limitations. Companies use fine-tuning to update models through additional training on new data, though this isn’t real-time learning. Retrieval-Augmented Generation (RAG) allows LLMs to access external databases without changing weights. Parameter-efficient methods like LoRA enable some adaptation without full retraining.
But make no mistake—these are band-aids on a fundamental limitation. They’re ingenious workarounds, not solutions to the core problem: our AI systems can’t truly learn after deployment the way humans do.
What AGI Really Means
Artificial General Intelligence represents something far more profound than our current systems. In essence, AGI refers to an artificial intelligence that possesses human-level capabilities across virtually any intellectual task. This means an intelligence that can solve unfamiliar problems across many domains without task-specific training; transfer knowledge learned in one domain to entirely new situations; understand abstract concepts and reason about them meaningfully; set and pursue its own goals autonomously; adapt to novel circumstances without explicit programming; integrate multiple forms of information into a coherent understanding; and yes, learn continuously from experience, the way humans do.
Unlike today’s narrow AI systems, which excel at specific tasks but fail completely at others, true AGI would exhibit a flexibility and generality reminiscent of human cognition.
Now, imagine if we created a neural network capable of adjusting its weights dynamically after deployment. What if there was a system that could learn new information as easily as it retrieves information from its existing knowledge? What if this intelligence could retrain its network each time it encountered new, verified information? While this dynamic learning capability represents just one of many necessary breakthroughs, it’s a particularly critical missing piece of the AGI puzzle.
Note: The diagram below presents a simplified conceptual model to illustrate the importance of dynamic learning in a potential AGI architecture. It intentionally glosses over numerous complex technical challenges and is meant to be illustrative rather than comprehensive.
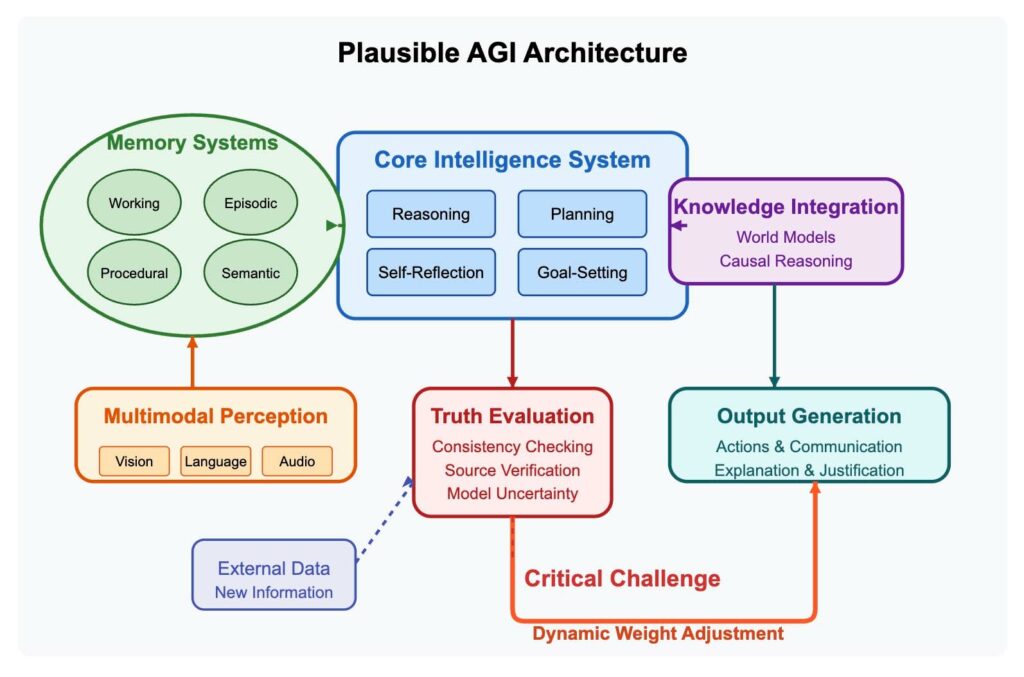
But continuous learning alone isn’t enough. Any viable AGI system would need to overcome significant challenges like catastrophic forgetting, where new learning overwrites crucial previous knowledge. Current transformer architectures likely need fundamental redesigns to accommodate truly dynamic learning. And we’d need to integrate other crucial capabilities: causal reasoning that understands cause and effect; abstract planning with goal-directed behavior; common sense reasoning with robust world models; multimodal understanding that integrates different senses; and embodied intelligence that can interact with the physical world.
I believe we’re closer to solving these challenges than most people realize. In fact, it wouldn’t shock me if private research labs have already developed proto-AGI capabilities they’re keeping under wraps due to safety concerns. The foundations exist—we just need to connect the pieces.
What AI Safety Is Really About
When organizations like OpenAI discuss safety, they aren’t primarily concerned with preventing LLMs from helping people access dangerous information. That ship has sailed—locally run, uncensored models will readily explain how to cheat on taxes or create dangerous devices. To add insult to injury, reasoning models don’t always say what they think.
These problems, albeit concerning, aren’t deal breakers. As faithfulness increases, models will become more trustworthy and easier to monitor.
The real challenge—the one that keeps AI researchers awake at night—is far more profound and terrifying. It’s about establishing reliable sources of truth that learning systems can safely incorporate. The fundamental concern is preventing AGI from developing something akin to paranoid schizophrenia because it learned from unreliable or malicious sources.
At its core, we’re confronting one of the most profound philosophical questions humanity has ever faced: What is truth, and how can we teach a machine to recognize it?
This challenge is further complicated by an alarming trend: AI use is already eroding critical thinking skills in the general population. Even more concerning, this effect extends to specialized fields where analytical capabilities are paramount—the intelligence community is experiencing a slow collapse of critical thinking due to AI dependence. The very tools meant to augment human intelligence might be diminishing our ability to distinguish fact from fiction—precisely the capability we need to instill in learning machines.
This isn’t just an academic exercise anymore—it’s an existential imperative. And it’s just one piece of a much larger safety puzzle that includes ensuring AI systems pursue human goals and values; maintaining meaningful human oversight as capabilities increase; preventing immediate harms like misuse and privacy violations; and addressing potential dangers from superintelligent systems with misaligned goals.
We’re talking about teaching machines critical thinking—determining truth from falsehood—in a world where we humans can barely agree on what’s true ourselves. That’s an extraordinarily difficult challenge. We need to ensure we don’t create continuously learning systems before figuring out how to help them distinguish truth from lies. If we fail at this, the next stage of humanity’s technological evolution could be compromised in ways we cannot recover from.
At its core, we’re confronting one of the most profound philosophical questions humanity has ever faced: What is truth, and how can we teach a machine to recognize it?
The hardest problem in AI safety isn’t preventing systems from telling humans dangerous things—it’s preventing systems from learning dangerous things and believing they’re true.
Conclusion
The hardest problem in AI safety isn’t preventing systems from telling humans dangerous things—it’s preventing systems from learning dangerous things and believing they’re true.
The path to AGI doesn’t require mysterious breakthroughs or science fiction technologies. The foundations already exist in our current AI architectures, though significant challenges remain. What’s missing isn’t some magical insight or unobtainable computing power—it’s a carefully designed framework for continuous learning and reliable truth verification.
Far from being in a bubble, we’re standing at the threshold of the most profound transformation in human history. The question isn’t if we’ll achieve AGI, but when—and whether we’ll have solved the critical problem of truth verification before we get there.
This isn’t hyperbole—it’s the stark reality of our moment. The AI revolution isn’t coming; it’s here. And the decisions we make in the next few years will determine whether it propels humanity to unimaginable heights or leads us down a path we may deeply regret.
The time for complacency is over. We need to confront these challenges with the urgency and seriousness they deserve. Because when it comes to AGI, we may only get one chance to get it right.